Ace Job Interviews with AI Interview Assistant
-
Get real-time AI assistance during interviews to help you answer the all questions perfectly.
-
Our AI is trained on knowledge across product management, software engineering, consulting, and more, ensuring expert answers for you.
-
Don't get left behind. Everyone is embracing AI, and so should you!
How It Works
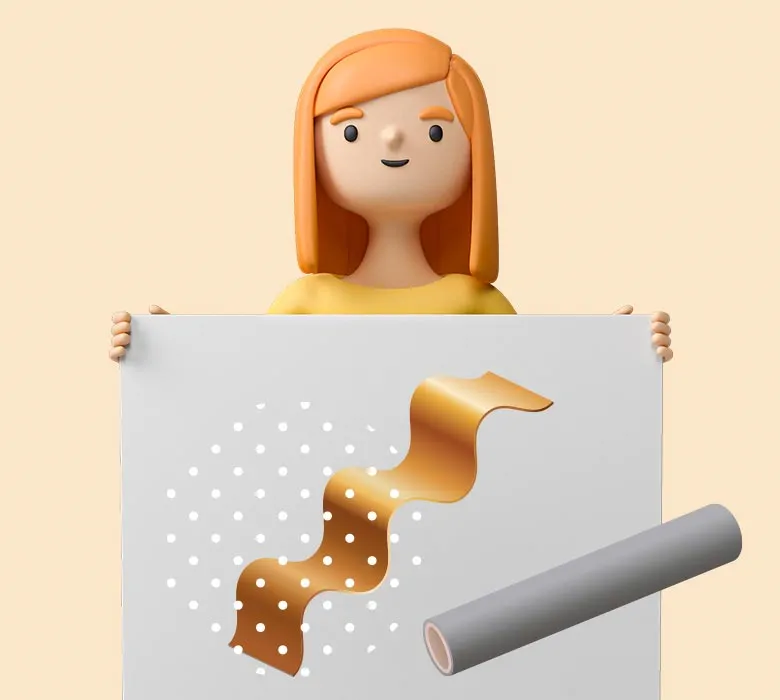
1. Upload your resume and job description
Give AI Interview Assistant everything it needs to know to help with your interview. Start by uploading your resume and the job descriptions for each of your interested positions. Behind the scenes, AI Interview Assistant will incorporate this with everything it knows about the relevant company positions to help formulate a plan for nailing your upcoming interviews.
2. Interview Assistant listens in and offers help
Once the interview starts, Ace Interview Assistant will listen in and provide you with personalized, accurate, and comprehensive response suggestions to interviewer questions. These answers will stream in fast in an easily digestible format that you can quickly pick up and use in your answers.
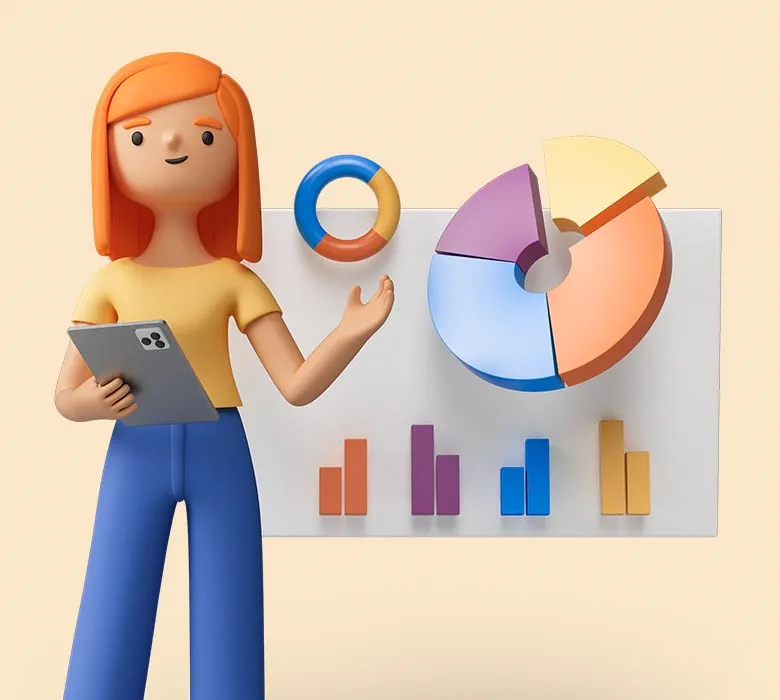
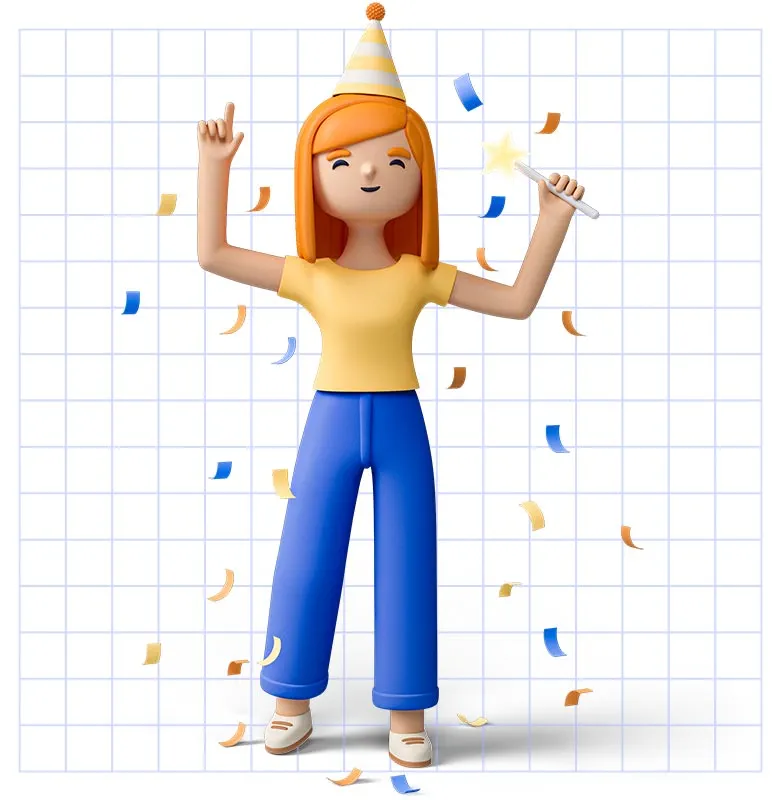
3. Offers start flowing in
Ace Interview Assistant will dramatically increase not just your success in passing tough interviews, but also surpass expectations and help you land higher level positions with more pay. Don't take chances. Even if you think you're a master interviewee, it doesn't hurt to have some super power help.
more offers
higher pay
TESTIMONIALS
Here's what some of our customers have to say...

I used to get so nervous during interviews. With Interview Assistant's help, I've been able to answer questions comprehensively and confidently. Just got a really good Senior SWE offer!

Jonathan G
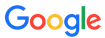

I used to struggle with PM product sense questions. I just can't think on the spot. Interview Assistant helped me crack them finally.

Brenda M


Got laid off last year from my consulting job, and couldn't land anything new. Things really turned around with Ace Interviews. It's like having God Mode turned on. Got an offer from my dream company!

David S
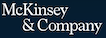

I've been a data scientist for 10+ years and couldn't get promoted, so thought I'd try interviewing elsewhere. Just landed a new position 2 levels higher. Ace Interview really helped me prepare.

Justin J
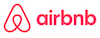
SUBSCRIPTION PLANS
Choose the best plan for your needs.
Trial
15 Minute Interview Sessions
- AI Interview Assistant
- No Support

Anually
Most Popular
Unlimited Interview Sessions
- AI Interview Assistant
- Priority Support
- Billed Annually - $288
Monthly
Unlimited Interview Sessions
- AI Interview Assistant
- Standard Support
- Billed Monthly - $89